
A multimodal image generation artificial intelligence (AI) model that supports each image generation and evaluation has emerged.
On the 14th (local time), Meta unveiled a multi-modal image generation artificial intelligence (AI) model, ‘CM3leon’, that creates images with text and describes images with text through its blog. No release date was mentioned.
Based on Meta, Chameleon used a pre-training method called masked token modeling, which divides image data into patches of a certain size and converts them into data represented by semantic tokens, as an alternative of the diffusion model utilized in existing image-generating AI models. .
This can be a commonly used method for constructing large language models comparable to ChatGPT. In that way, the chameleon learned to know patterns in images (image recognition) and create latest patterns (image creation).
After pre-training, Meta fine-tuned Chameleon to know complex prompts useful for spawning tasks.
Supervised Wonderful-Tuning makes it possible to perform a wide range of multimodal tasks, comparable to image captioning, visual query answering, text-based editing, and conditional image creation.
Map fine-tuning has also been applied to coach text generation models comparable to ChatGPT to great effect.
Particularly, Meta trained Chameleon using a dataset of thousands and thousands of images licensed from Shutterstock. Meta trained a chameleon with 7 billion parameters on a dataset of small text tokens of about 3 billion.
Nevertheless, various vision language tasks comparable to answering visual questions and dealing with subtitles were performed without problems. Chameleon recorded the next level of performance with 5x fewer computations and a smaller training dataset than existing AI models.
This approach allows Chameleon to generate the precise images you wish. For instance, I used to be in a position to successfully create objects based on complex descriptions comparable to ‘a small cactus wearing a straw hat and neon sunglasses within the Sahara’.
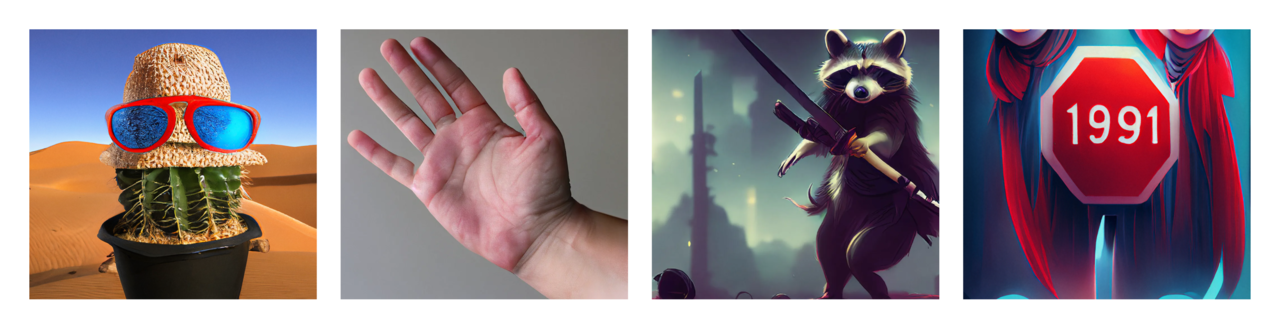
Also, given a picture and text prompt, the identical model might be used to edit the image in response to the text’s instructions.
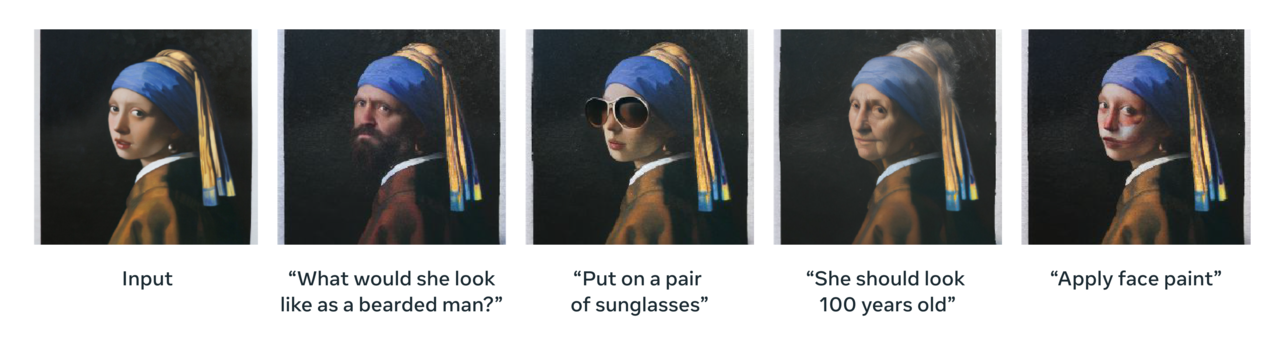
Chameleon also can generate short or long captions based on various prompts and answer questions on images.
For instance, for a picture of a ‘dog holding a stick’, if you happen to ask ‘what’s the dog carrying?’, the model will answer ‘stick’, and if you happen to ask ‘describe the given image in great detail’, the model will answer ‘ On this image there’s a dog with a stick in its mouth. There’s grass on the surface. There’s a tree within the background of the image’.
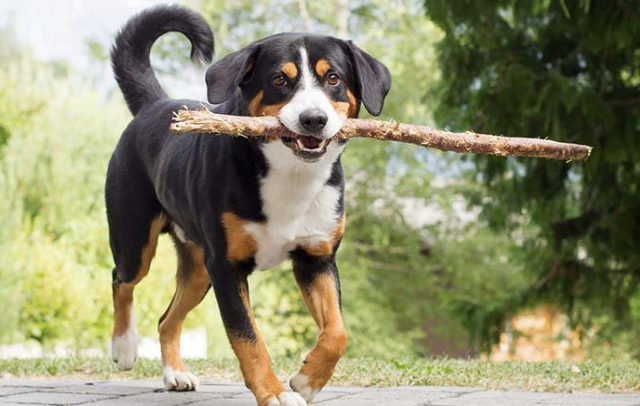
It understands and interprets textual instructions in addition to structural or layout information provided as input, allowing image editing to be visually consistent and in context. For instance, given a textual description of a picture’s bounding box segmentation, it creates a picture.
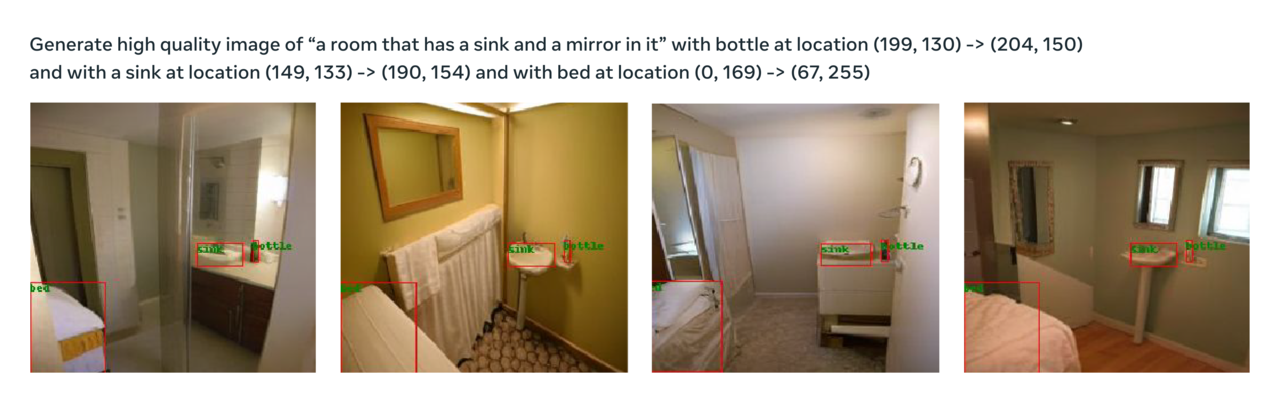
A model can create a picture given a picture that comprises only segmentation with out a description of the article.
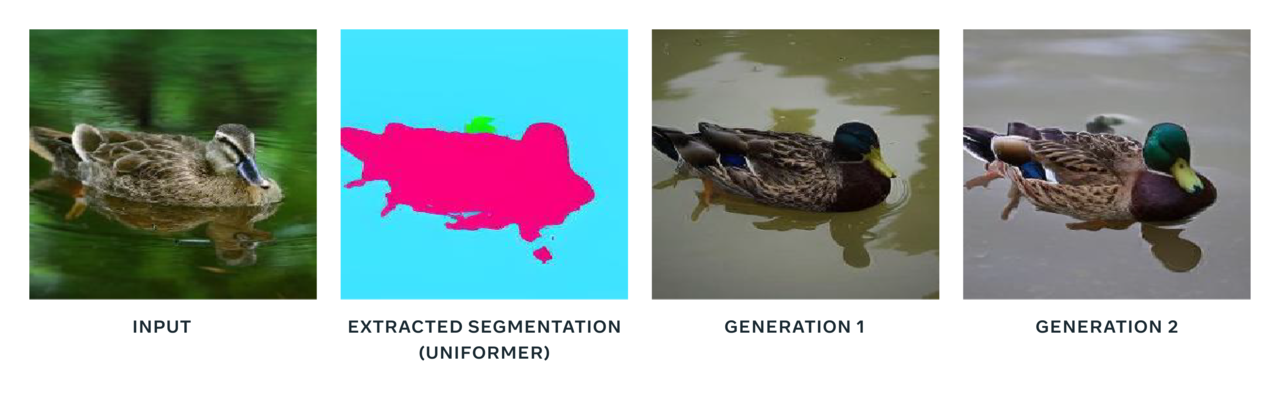
As well as, individually learned steps might be added to represent high-resolution images.
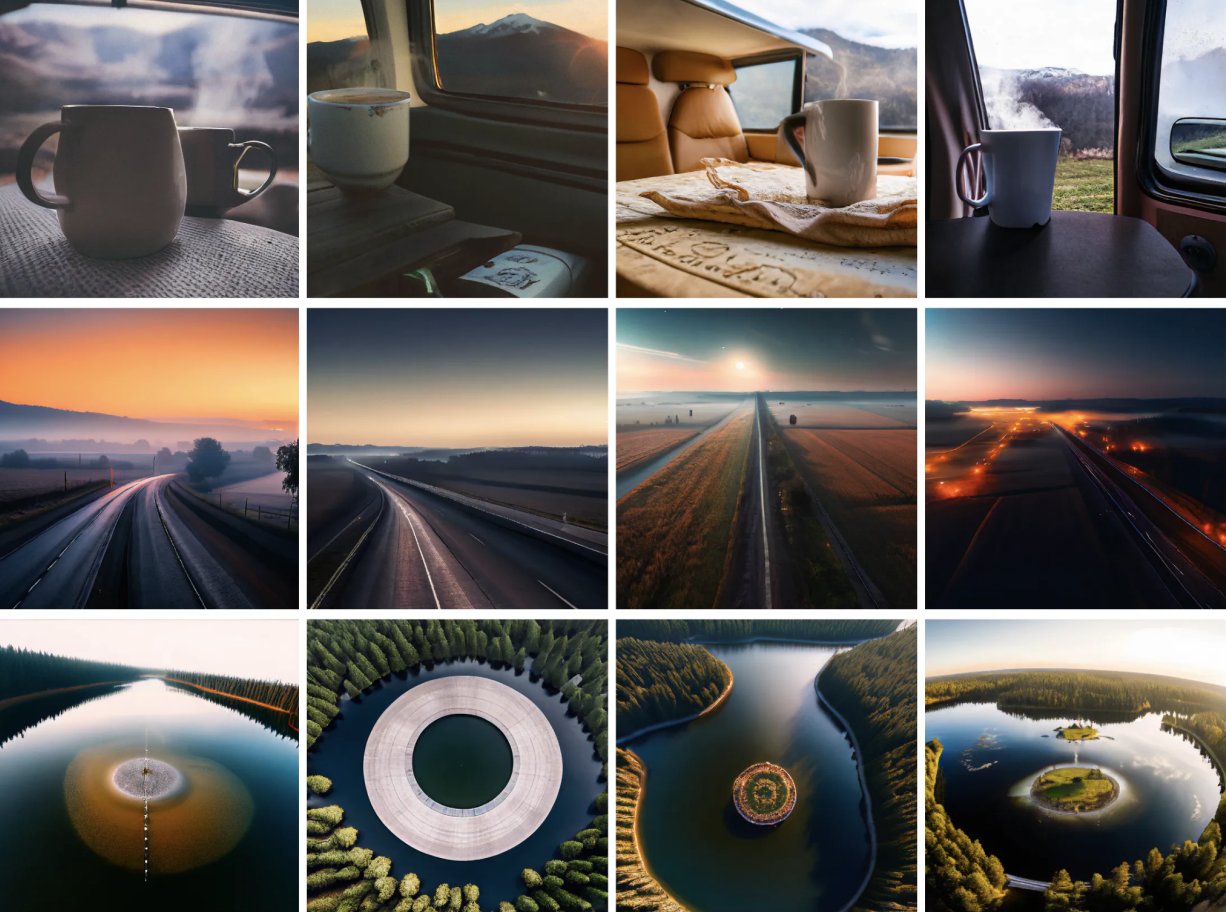
“We imagine the strong performance of Chameleon on a wide range of tasks is a step towards higher fidelity image generation and understanding,” said Meta. “Models like Chameleon will ultimately serve to boost creativity and higher applications within the Metaverse. It could actually help,” he said.
Reporter Park Chan cpark@aitimes.com
… [Trackback]
[…] Info on that Topic: bardai.ai/artificial-intelligence/meta-unveils-multimodal-image-creation-ai-chameleon/ […]
… [Trackback]
[…] Read More to that Topic: bardai.ai/artificial-intelligence/meta-unveils-multimodal-image-creation-ai-chameleon/ […]
instrumental music
… [Trackback]
[…] Information on that Topic: bardai.ai/artificial-intelligence/meta-unveils-multimodal-image-creation-ai-chameleon/ […]
… [Trackback]
[…] Find More Information here to that Topic: bardai.ai/artificial-intelligence/meta-unveils-multimodal-image-creation-ai-chameleon/ […]
… [Trackback]
[…] Information to that Topic: bardai.ai/artificial-intelligence/meta-unveils-multimodal-image-creation-ai-chameleon/ […]
… [Trackback]
[…] Info to that Topic: bardai.ai/artificial-intelligence/meta-unveils-multimodal-image-creation-ai-chameleon/ […]
… [Trackback]
[…] Read More here on that Topic: bardai.ai/artificial-intelligence/meta-unveils-multimodal-image-creation-ai-chameleon/ […]