
The Korea Advanced Institute of Science and Technology (KAIST, President Lee Kwang-hyung) announced on the fifth that the research team led by Professor Seung-Hoon Hong of the Department of Computer Science won the Best Paper Award on the International Society for Expressive Learning 2023 (ICLR 2023), a global machine learning conference.
ICLR 2023 is one of the vital prestigious conferences in the sphere of artificial intelligence (AI), rating first in the sphere of machine learning based on the Google Scala h-5 index and rating ninth amongst publications in all scientific fields. This 12 months’s Best Paper Award was given to the highest 4 papers out of a complete of 1574 papers.
He explained that Professor Hong’s team was the primary Korean to win the ICLR Best Paper Award, and that it was also the primary case of winning the Best Paper Award at a significant machine learning society for a research centered on a domestic institution.
A research team consisting of Ph.D. student Dongkyun Kim (first writer), Ph.D. student Jinwoo Kim, Ph.D. student Cho Sung-woong, and Dr. Chong Luo from Microsoft Research Asia on the Department of Computer Science at KAIST solved the ‘pixel labeling problem’, a key research topic in the sphere of computer vision, with a small amount of information. We proposed a Visual Token Matching technique, a general-purpose methodology that may solve a wide selection of problems.
Pixel labeling is an idea that encompasses core problems in computer vision, corresponding to object detection, object segmentation, pose estimation, depth estimation, and 3D reconstruction. Prior to now decade, progress has been made within the detailed problem of pixel labeling with neural network-based machine learning methodologies, but there’s a limitation that requires greater than lots of of hundreds of coaching data.
Professor Seung-Hoon Hong’s research team has developed a general-purpose Fewshot learning technique that may learn and infer with lower than dozens of information for pixel labeling problems, and may achieve similar or superior performance with lower than 0.01% data in comparison with existing methods in quite a few pixel labeling problems. proved that it may
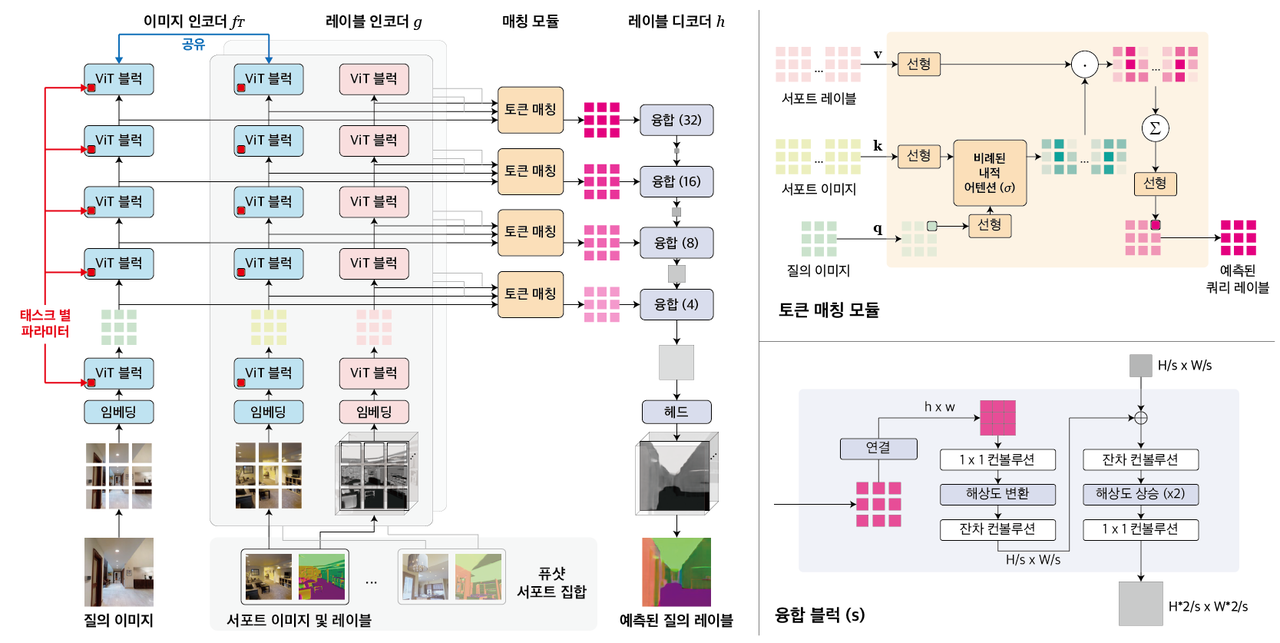
Professor Hong said that he expects this research to be a breakthrough in applying computer vision technology in various domains where learning data collection is a bottleneck, corresponding to medical imaging.
Doctoral student Dongkyun Kim, who led the research, has been repeatedly researching a general-purpose machine learning methodology that may learn with a small amount of information, and has published a study that serves because the theoretical basis for this research in ICLR. Doctoral course Dongkyun Kim won the silver prize on the Samsung Human Tech Thesis Awards for this research.
Professor Hong Seung-hoon of the Department of Computer Science at KAIST said, “It’s an honor to receive this award, and I can be completely satisfied if this award would help domestic machine learning researchers turn into more confident and help more difficult research come out in Korea.”
Reporter Lim Dae-jun ydj@aitimes.com
relaxing piano music