
Within the AI ecosystem, diffusion models are establishing the direction and pace of technological advancement. They’re revolutionizing the best way we approach complex generative AI tasks. These models are based on the mathematics of gaussian principles, variance, differential equations, and generative sequences. (We’ll explain the technical jargon below)
Modern AI-centric products and solutions developed by Nvidia, Google, Adobe, and OpenAI have put diffusion models at the middle of the limelight. DALL.E 2, Stable Diffusion, and Midjourney are distinguished examples of diffusion models which might be making rounds on the web recently. Users provide an easy text prompt as input, and these models can convert them into realistic images, resembling the one shown below.
A picture generated with Midjourney v5 using input prompt: vibrant California poppies. Source: Midjourney
Let’s explore the basic working principles of diffusion models and the way they’re changing the directions and norms of the world as we see it today.
What Are Diffusion Models?
In keeping with the research publication “Denoising Diffusion Probabilistic Models,” the diffusion models are defined as:
“A diffusion model or probabilistic diffusion model is a parameterized Markov chain trained using variational inference to supply samples matching the info after finite time”
Simply put, diffusion models can generate data much like those they’re trained on. If the model trains on images of cats, it might probably generate similar realistic images of cats.
Now let’s try to interrupt down the technical definition mentioned above. The diffusion models take inspiration from the working principle and mathematical foundation of a probabilistic model that may analyze and predict a system’s behavior that varies with time, resembling predicting stock market return or the pandemic’s spread.
The definition states that they’re parameterized Markov chains trained with variational inference. Markov chains are mathematical models that outline a system that switches between different states over time. The present state of the system can only determine the probability of transitioning to a particular state. In other words, the present state of a system holds the possible states a system can follow or acquire at any given time.
Training the model using variational inference involves complex calculations for probability distributions. It goals to seek out the precise parameters of the Markov chain that match the observed (known or actual) data after a particular time. This process minimizes the worth of the model’s loss function, which is the difference between the anticipated (unknown) and observed (known) state.
Once trained, the model can generate samples matching the observed data. These samples represent possible trajectories or state the system could follow or acquire over time, and every trajectory has a unique probability of happening. Hence, the model can predict the system’s future behavior by generating a variety of samples and finding their respective probabilities (likelihood of those events to occur).
The way to Interpret Diffusion Models in AI?
Diffusion models are deep generative models that work by adding noise (Gaussian noise) to the available training data (also often called the forward diffusion process) after which reversing the method (often called denoising or the reverse diffusion process) to recuperate the info. The model step by step learns to remove the noise. This learned denoising process generates recent, high-quality images from random seeds (random noised images), as shown within the illustration below.

Reverse diffusion process: A loud image is denoised to recuperate the unique image (or generate its variations) via a trained diffusion model. Source: Denoising Diffusion Probabilistic Models
3 Diffusion Model Categories
There are three fundamental mathematical frameworks that underpin the science behind diffusion models. All three work on the identical principles of adding noise after which removing it to generate recent samples. Let’s discuss them below.

A diffusion model adds and removes noise from a picture. Source: Diffusion Models in Vision: A Survey
1. Denoising Diffusion Probabilistic Models (DDPMs)
As explained above, DDPMs are generative models mainly used to remove noise from visual or audio data. They’ve shown impressive results on various image and audio denoising tasks. For example, the filmmaking industry uses modern image and video processing tools to enhance production quality.
2. Noise-Conditioned Rating-Based Generative Models (SGMs)
SGMs can generate recent samples from a given distribution. They work by learning an estimation rating function that may estimate the log density of the goal distribution. Log density estimation makes assumptions for available data points that its a component of an unknown dataset (test set). This rating function can then generate recent data points from the distribution.
For example, deep fakes are notorious for producing fake videos and audios of famous personalities. But they’re mostly attributed to Generative Adversarial Networks (GANs). Nonetheless, SGMs have shown similar capabilities – at times outperform – in generating high-quality celebrity faces. Also, SGMs can assist expand healthcare datasets, which usually are not available in large quantities as a result of strict regulations and industry standards.
3. Stochastic Differential Equations (SDEs)
SDEs describe changes in random processes concerning time. They’re widely utilized in physics and financial markets involving random aspects that significantly impact market outcomes.
For example, the costs of commodities are highly dynamic and impacted by a variety of random aspects. SDEs calculate financial derivatives like futures contracts (like crude oil contracts). They’ll model the fluctuations and calculate favorable prices accurately to provide a way of security.
Major Applications of Diffusion Models in AI
Let’s have a look at some widely adapted practices and uses of diffusion models in AI.
High-Quality Video Generation
Creating high-end videos using deep learning is difficult because it requires high continuity of video frames. That is where diffusion models turn out to be useful as they will generate a subset of video frames to fill in between the missing frames, leading to high-quality and smooth videos with no latency.
Researchers have developed the Flexible Diffusion Model and Residual Video Diffusion techniques to serve this purpose. These models can even produce realistic videos by seamlessly adding AI-generated frames between the actual frames.
These models can simply extend the FPS (frames per second) of a low FPS video by adding dummy frames after learning the patterns from available frames. With almost no frame loss, these frameworks can further assist deep learning-based models to generate AI-based videos from scratch that appear to be natural shots from high-end cam setups.
A wide selection of remarkable AI video generators is out there in 2023 to make video content production and editing quick and simple.
Text-to-Image Generation
Text-to-image models use input prompts to generate high-quality images. For example, giving input “red apple on a plate” and producing a photorealistic image of an apple on a plate. Blended diffusion and unCLIP are two distinguished examples of such models that may generate highly relevant and accurate images based on user input.
Also, GLIDE by OpenAI is one other widely known solution released in 2021 that produces photorealistic images using user input. Later, OpenAI released DALL.E-2, its most advanced image generation model yet.
Similarly, Google has also developed a picture generation model often called Imagen, which uses a big language model to develop a deep textual understanding of the input text after which generates photorealistic images.
We’ve got mentioned other popular image-generation tools like Midjourney and Stable Diffusion (DreamStudio) above. Have a have a look at a picture generated using Stable Diffusion below.
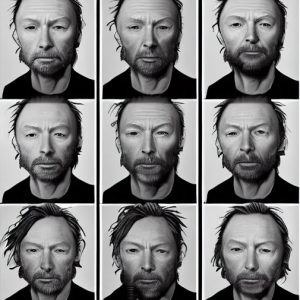
A picture created with Stable Diffusion 1.5 using the next prompt: “collages, hyper-realistic, many variations portrait of very old thom yorke, face variations, singer-songwriter, ( side ) profile, various ages, macro lens, liminal space, by lee bermejo, alphonse mucha and greg rutkowski, greybeard, smooth face, cheekbones”
Diffusion Models in AI – What to Expect within the Future?
Diffusion models have revealed promising potential as a strong approach to generating high-quality samples from complex image and video datasets. By improving human capability to make use of and manipulate data, diffusion models can potentially revolutionize the world as we see it today. We are able to expect to see much more applications of diffusion models becoming an integral a part of our day by day lives.
Having said that, diffusion models usually are not the one generative AI technique. Researchers also use Generative Adversarial Networks (GANs), Variational Autoencoders, and flow-based deep generative models to generate AI content. Understanding the basic characteristics that differentiate diffusion models from other generative models can assist produce simpler solutions in the approaching days.
To learn more about AI-based technologies, visit Unite.ai. Take a look at our curated resources on generative AI tools below.