
It has been identified that the rapidly growing artificial intelligence (AI) model is threatened by a scarcity of knowledge. The reason is that there might be limitations in improving AI model performance inside two years with only the info that currently exists on the Web.
The Wall Street Journal (WSJ) reported on the first (local time) that technologies corresponding to OpenAI's 'GPT-4' and Google's 'Gemini' are facing a possible data shortage.
In accordance with this, as the dimensions of huge language models (LLMs) grows, unprecedented demand for data is happening within the industry. AI corporations are expressing the opinion that inside the subsequent two years, demand for high-quality text data will exceed supply, potentially hindering AI development.
Epoch researcher Pablo Virarobos said, “In accordance with the 'Chinchilla scaling law' amongst computer science principles, an AI system corresponding to 'GPT-5' would require 60 to 100 trillion data tokens if it follows its current growth trajectory.” “This might exceed all currently available high-quality text by a minimum of 10 to twenty trillion pieces of knowledge.”
The chinchilla scaling law originated from the outcomes of an experiment conducted in 2022 that compared the performance of the Chinchilla model with 70 billion parameters and the Gopher model with 280 billion parameters. We estimated the quantity of coaching data needed for a selected parameter model to realize optimal performance. Within the case of the chinchilla model, even though it is simply 1 / 4 of the dimensions of the gopher, it showed higher performance since it was trained with 4 times more data.
In accordance with the law inferred here, a model with 530 billion parameters requires training data price 11 trillion tokens. Due to this fact, the conclusion is that within the case of GPT-5, which is anticipated to have 2 trillion parameters, learning greater than 60 trillion tokens is essential.
Nevertheless, the issue is that there will not be much usable data among the many vast amount of knowledge on the Web. Most of them are of low quality or have limited access as a result of copyright issues.
To resolve this problem, researchers are exploring all types of methods, including discovering recent data sources corresponding to synthetic data, YouTube videos, and community posts corresponding to Reddit, and improving learning efficiency through ML techniques corresponding to curriculum learning. Nevertheless, synthetic data also carries the danger of 'model collapse', which suddenly reduces model performance.
Regarding this, Airi Morkos, founder and CEO of Daytology AI, said, “Data scarcity continues to be an unexplored research area,” and added, “That is the hidden secret of deep learning. Identical to checking if spaghetti is cooked by throwing it on the wall, you may't know unless you truly shut up.” “It’s not an issue,” he said.
WSJ also identified problems within the ecosystem, corresponding to a scarcity of infrastructure supply corresponding to AI chips and power, and a decline in web and app traffic as a result of the activation of AI chatbots.
Sam Altman, CEO of Open AI, also expressed similar thoughts a while ago. “I feel we're at the tip of the era of the large model,” he said. “So we're going to make it higher in other ways.”
For that reason, it is anticipated that in the long run, specialized models which have intensively learned domain-specific knowledge will dominate slightly than large general-purpose models corresponding to GPT-4.
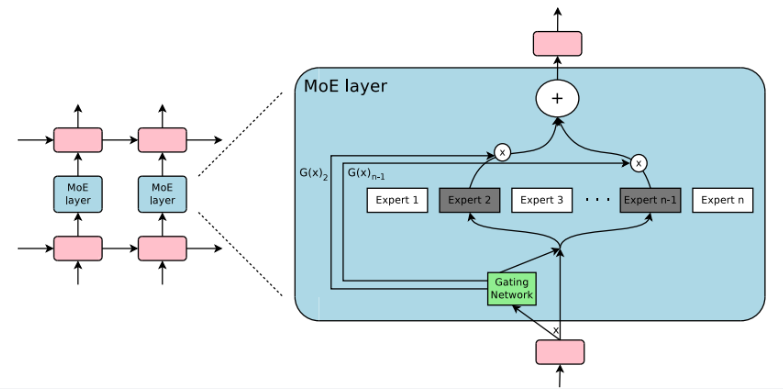
In truth, in recent LLM-related research and product launch trends, there are various cases of mixing multiple small models and switching them based on Curie slightly than a single large model. As well as, even within the case of a single model, the ‘mixture of experts (MoE)’ method, which divides a single model into several skilled models, is becoming a trend.
After OpenAI was known to have introduced this method in earnest in GPT-4 last yr, Mistral AI introduced it in 'Mixtral 8x7B' and made it extremely popular. In truth, it is a technique that focuses on efficiency slightly than performance.
Among the many models released previously week, major models corresponding to AI21 Labs' 'Jamba', Sambanova's 'Samba-CoE v0.2', and Databricks' 'DBRX' all adopted the MoE method. Even xAI's 'Grock' was revealed to be an MoE method.
Reporter Lim Da-jun ydj@aitimes.com