
A synthetic intelligence (AI) tool has been developed that generates synthetic data from situations which are difficult to gather in point of fact, similar to a highway where a taxi overturns across two lanes or a pedestrian dressed as a dinosaur jumps into the roadway.
It is feasible to conduct AI system training more effectively by generating data that’s mandatory for training AI systems applied to self-driving cars or drones, but is definitely insufficient.
TechCrunch reported on the nineteenth (local time) that US start-up Parallel Domain has released a latest API called ‘Data Lab’ that may generate such synthetic data.
Data Lab is a tool that uses generative AI to create scenes depicting specific situations. By entering easy prompts similar to ‘make this image appear like a snowstorm’ or ‘drop raindrops on the lens’, latest objects are added to the present image or transformed to suit the situation.
Parallel Domain used its own synthetic data generation engine called ‘Reactor’ for this purpose. Reactor utilizes fine-tuned models and 3D simulations of “Stable Diffusion,” an image-generating AI, to construct detailed, realistic synthetic data sets. Generative AI can generate various scenarios and objects, and 3D simulation adds to the physical realism of the scene.
You possibly can create synthetic data by installing the Data Lab API from GitHub and writing Python code.
Using Python, users have the flexibleness to construct synthetic datasets by choosing different parameters similar to location (San Francisco, Tokyo), environment (city, suburbs, highway), weather conditions, and agent distribution (pedestrians, vehicles).
Once the essential data set is configured, users can use Reactor to further enhance the range and realism of synthetic data.
Using natural language prompts, users can add different objects and scenarios to the scene, similar to ‘garbage can’, ‘cardboard box stuffed with sunglasses’, ‘orange crate’, ‘stroller’, etc.
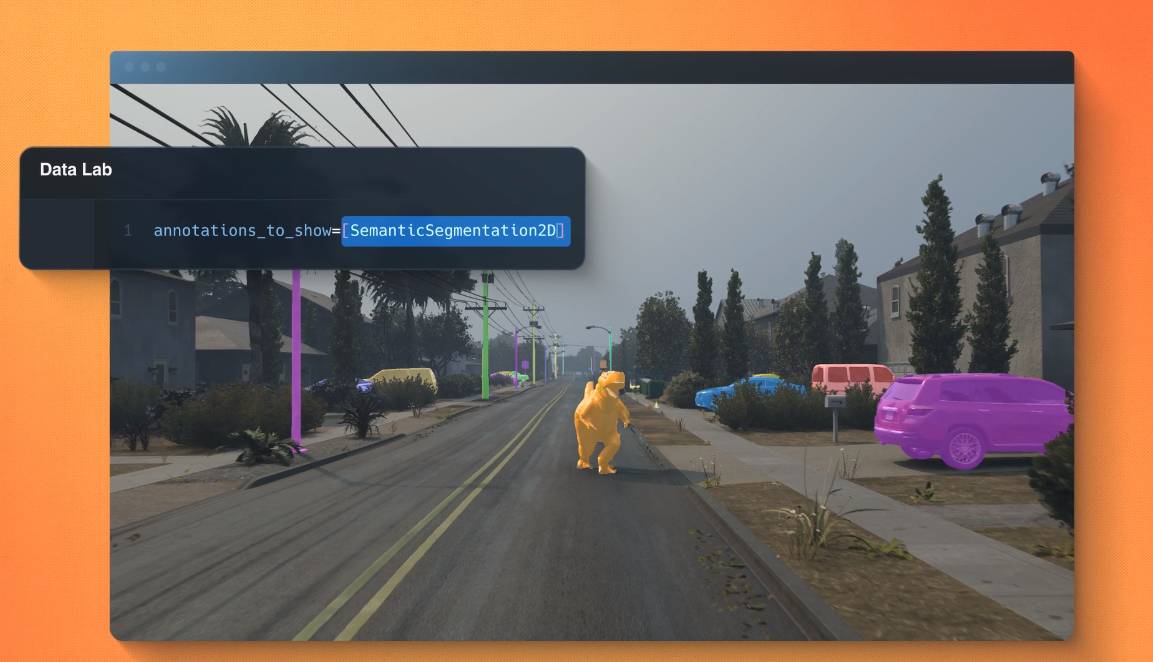
As well as, Reactor can generate synthetic data with essential annotations similar to bounding boxes and semantic segmentation, which may significantly speed up AI model training and testing.
Parallel Domain seeks to advance autonomous driving system technology and advanced driver assistance systems (ADAS) with data labs. The goal is to assist autonomous driving, drone and robotics firms construct large datasets more efficiently.
“It takes weeks or months to construct a dataset, however the Data Lab API allows us to construct latest datasets in near real time,” said Parallel Domain CEO Kevin McNamara. “Training relies on synthetic data fairly than real data. “This result was even higher,” he stressed.
Reporter Park Chan cpark@aitimes.com