MLCommons, a man-made intelligence (AI) engineering consortium, recently showed the outcomes of ‘MLPerf Inference’, which measures the performance of hardware infrastructure constituting data centers, with Nvidia products showing excellent overall performance, and other corporations’ products that participated within the test. Usually, the performance was also significantly improved.
ML Puff is a benchmark test standard prepared by ML Commons. ML Puff reasoning is carried out in the shape of participating corporations testing their products based on this standard and submitting the outcomes to ML Commons.
Nevertheless, for the reason that test only proceeds with the items that the participating corporations want, it may only be evaluated by itself, and a full comparative evaluation is unattainable.
ML Commons announced the benchmark results of ‘ML Puff Inference 3.0’ on its blog on the fifth (local time).
MLPerf Inference 3.0 provides about 6700 inference performance results and about 2400 power efficiency measurements. The outcomes of this benchmark showed an influence efficiency increase of greater than 50% and a performance improvement of greater than 60% in some benchmark tests.
Alibaba, Asus, MS, Ctuning, Desi, Dell, Gigabyte, H3C, HPE, Inspur, Intel, Cry, Lenovo, Moffett, Nettrix, Newchips, Neural Magic, Nvidia, Qualcomm, Quanta Cloud , Revolt, Cima, Supermicro, VMware, and Xfusion participated.
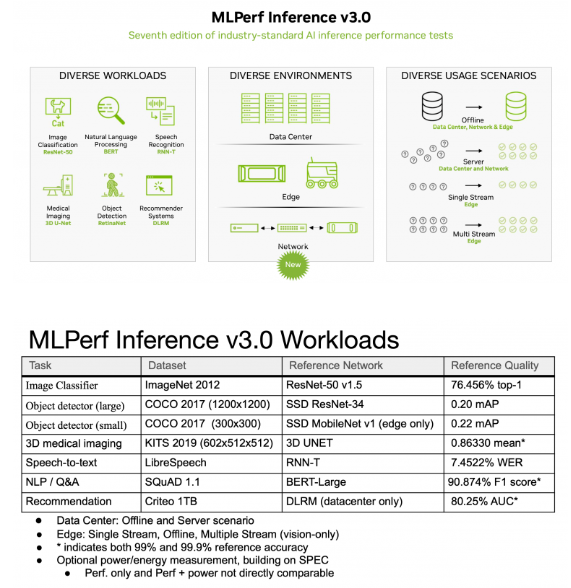
The MLPerf Inference 3.0 test can run benchmarks against options consisting of image classification, object detection, suggestion, speech recognition, natural language processing and 3D medical imagery. The inference performance of the test participants’ data center and edge systems is measured using the info set and AI model specified for every option.
The most important trend on this benchmark test is that it achieved a remarkable performance improvement across the board.
Intel’s latest accelerator AMX, for instance, wasn’t as fast as GPU-based systems, but was an improvement over previous-generation products.
As well as, Qualcomm submitted that an information center server platform equipped with 18 ‘Cloud AI 100’ chips recorded excellent offline performance and power efficiency. Qualcomm claims to have improved performance by as much as 86% and power efficiency by as much as 52% for the reason that first MLPuff 1.0 test through software optimization.
VMware, working with Dell and Nvidia, virtualized the Nvidia Hopper system and reported that it achieved 94 percent of 205 percent of bare metal performance while using only 16 CPU cores out of 128 logical CPU cores. The remaining 112 CPU cores were freed up for other workloads via virtualization without affecting the performance of the machine running the inference workload.
Meanwhile, the most important trend in AI inference immediately has been large-scale inference in LLMs like ChatGPT. The GPT-class model was not included within the ML Puff benchmark suite, but might be included within the Q2 training benchmark.
Chan Park, cpark@aitimes.com